How the Pandemic Has Highlighted the Need for the Next Generation of Natural Catastrophe Impact Modeling
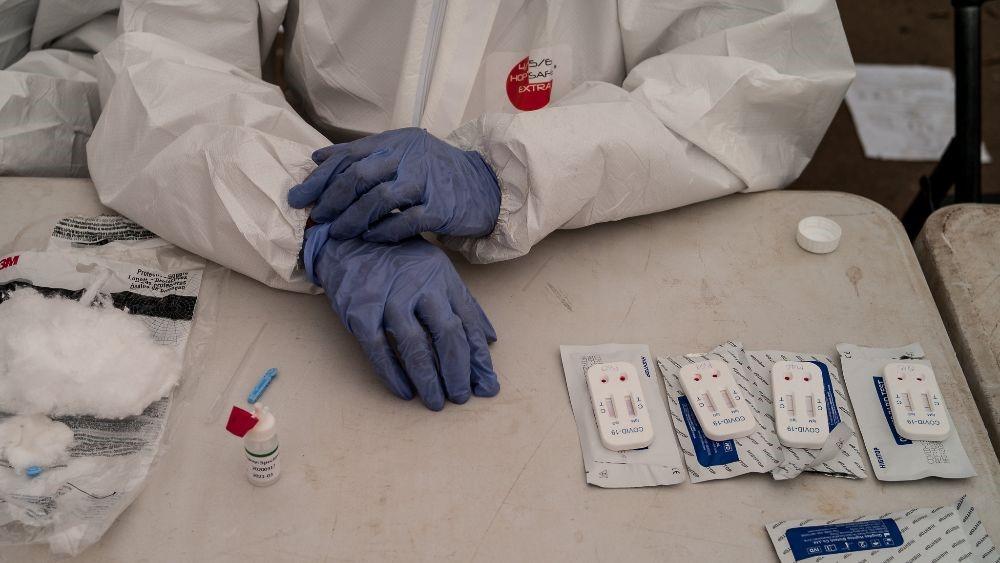
Over the last decade, the World Bank’s Disaster Risk Finance and Insurance Program (DRFIP) has worked with low- and middle-income countries to build their financial resilience to disasters which contributes to the World Bank’s twin goals of poverty reduction and shared prosperity. To help a country become more financially resilient to the risks it faces, we first consider what the risks are, how likely they are to materialize, and what social and economic losses they would cause. We can answer some of these questions with natural catastrophe models, as used by the insurance industry, to quantify the expected levels of losses from different types of physical disasters and the frequency with which they are likely to occur.
Given the level of vulnerability in developing countries, our focus has primarily been on physical events, i.e., tropical cyclones, earthquakes, droughts, and floods. However, when the COVID-19 pandemic struck, it was clear that the social and economic implications would be significant. It was therefore important to learn more about how pandemics are modeled, and what lessons can help expand the risk modeling tool kit available to our clients, so they could better assess and ultimately manage the compounding effects of such risks.
We partnered with Metabiota, an epidemiological modeling firm, to learn how they model the novel coronavirus, including any key assumptions and limitations. Together, we reviewed the modeled scenarios for potential COVID-19 infection numbers in several countries, and the potential impact on these numbers of different interventions and other compounding events (for example, the impact on case numbers if a Category 5 hurricane occurred at the same time as a peak in COVID-19 cases).
Below, we summarize what we learned about the differences between natural catastrophe modeling and epidemiological modeling. We focus on the use cases of the models when an event is ongoing as opposed to the approaches for developing risk profiles.
During an event the structure of a natural catastrophe model is very different from an epidemiological model.
Disaster risk finance focuses on pre-arranged financial solutions; rather than monitoring real-time events, it typically relies on a stochastic event set and associated modeled probabilities of certain levels of loss to understand a country’s risk. Natural catastrophe models are primarily designed for the purpose of understanding risk, and do not predict the unfolding of events in real time (they are not forecasting models). Instead, they use meteorological agency forecasts within their catastrophe modeling framework to provide indications of potential losses from an event as it unfolds.
Tropical cyclone events, for example, are a frequent weather phenomenon; but if we look at a specific hurricane heading toward the Caribbean, the exact trajectory is often very uncertain, even within days or hours from landfall. Prior to landfall, natural catastrophe modeling firms aim to help their clients understand the range of potential losses by selecting the stochastic events that are most similar to the forecasted trajectory and intensity of the storm as predicted by weather forecasting agencies, such as the European Centre for Medium-Range Weather Forecasts (ECMWF) and National Oceanic and Atmospheric Administration (NOAA). The modeling firms may also use the ensemble of forecast tracks provided by these agencies as input to their model to calculate the range of possible losses from the forecasted tracks. This range can be large when the storm is still far from landfall; for example, one of the physically possible forecast tracks may indicate that the storm will impact a major city and cause a higher loss, while another may suggest landfall in a relatively sparsely populated location, leading to a smaller loss.
As the storm gets closer to land and the meteorological agencies provide updated forecasts (see figure 1), the uncertainty of the location and intensity of landfall decreases, and catastrophe modeling firms typically aim to refine their selection of stochastic events. Similarly, the range of the projected losses from the ensemble of forecast tracks will also likely become smaller.
Figure 1: Forecast tracks for Cyclone Harold from several forecast agencies, April 2020
Source: National Center for Atmospheric Research (NCAR)
Epidemiological models often use a compartmentalized model. Susceptibility, exposure, infectivity, and recovery—abbreviated as SEIR—are “disease states” and allow for the inclusion of risk factors (i.e., access to hospitals, population density, and population age) at different stages of a disease life cycle. One of the key parameters in the model is the effective reproductive number (R) of the disease at any given point, and the starting proportion of the population in various risk brackets. From these parameters, the daily rate of new infections, hospitalizations, and fatalities are calculated as a time series over the assumed life cycle of the event.
Figure 2: Compartmental SEIR model structure
There are four key differences in the modeling approaches of natural catastrophe and epidemiological models:
1. Time scales are inherently different.
Earthquakes are over in a matter of seconds to minutes, and tropical cyclones have a lifetime of days to weeks, but epidemiological events can last years. The time scale of COVID-19 is already measured in years, which makes it fundamentally much harder to model the trajectory of possible outcomes.
Models for slow-onset disasters such as drought or food insecurity have not historically been produced by risk modeling vendors, perhaps due to the difficultly of assessing the vulnerability and impact. However, these events have significant social and economic impacts in developing countries, particularly Sub-Saharan Africa, and better modeling techniques are needed to understand the protection gaps. Given that time scales of disease outbreaks are more in line with slow-onset disasters, we may be able to learn how to model such events from the pandemic modeling community.
2. The cone of uncertainty around a pandemic does not necessarily decrease with time.
Typically, for the modeling of a tropical cyclone event, we would expect the range of likely outcomes to decrease as a storm’s intensity and direction become more certain, with the final days’ forecasts being subsets of the earlier forecasts. As a pandemic unfolds, we hope to learn more on the infectivity and recovery rates; but the impacts also depend on many exogenous variables, such as a government’s decision on nonpharmaceutical interventions or the availability and efficacy of a vaccine. Thus the uncertainty in potential trajectories can remain very large.
3. There is no geographic boundary in pandemic modeling.
While there is growing discussion in the catastrophe modeling community about the potential disadvantages of independently modeling areas of the world and ignoring potential correlations between areas, an individual event is always a local phenomenon, and its direct losses are by and large independent from the rest of the world. The COVID-19 outbreak has highlighted that the actions of one country directly affect another—for example, through closing of borders or differing lockdown rules; and pandemic models need to account for this. Mobility data are used in the model to assess the public’s adherence to government-mandated social-distancing measures as well as to compare the relative effectiveness of the measures and the trend over time.
4. Robust pandemic modeling involves understanding the actions of individuals.
Likely many of us have heard the famous quote, “A butterfly can flutter its wings over a flower in China and cause a hurricane in the Caribbean.” But clearly neither natural catastrophe models nor the most sophisticated weather forecasting models actually include what a butterfly is doing on a given day in China.
However, what about the actions of a single person in the world during a global pandemic? There have been several stories in the media about the actions of individuals leading to COVID-19 outbreaks, which have then resulted in “circuit breaker” lockdowns in cities or even border closures. These interventions have a large impact on the economy and ultimately the economic loss that countries face because of COVID-19.
Currently, epidemiological models do not go so far as to estimate the economic losses of such events, but they do consider that individuals’ willingness to adopt nonpharmaceutical interventions (such as social distancing) has an impact on curbing the rate of infections. Attempting to model the actions of people presents a far more daunting task than what natural catastrophe modeling aims to achieve.
Photo Credit: UN Photo/OCHA/Mark Garten
Nonmodeled losses are key to understanding the full impact of pandemics and natural catastrophes on financial, economic, and welfare impacts.
Epidemiological modeling can provide information only on the spread and severity of a disease. Economic loss calculations come in at a secondary stage—for example, in considering the impact of the disease on the labor force (if many people take sick leave) or the impact of mitigation measures, such as school and business closures. The disease itself does not impact physical infrastructure in the same way that a natural catastrophe might, but it does affect the ability of people to participate in the economy, in a variety of different ways.
Following a large physical event, catastrophe modeling firms provide estimates of the industry loss based on their modeled loss. In order to estimate their total losses, insurers must then consider the nonmodeled losses they will incur; these are the potential sources of insurance loss that may arise as a result of the event but that existing catastrophe models do not explicitly cover. Nonmodeled losses could include loss adjustment expenses, regulatory intervention, workers compensation, or contingent business interruption (which is the decreased ability of suppliers affected by a disaster to meet contracts to their customers around the world). Many of these nonmodeled losses are the very types of losses we need to be able to quantify to understand how large-scale pandemics may impact a country’s economy.
We recognize that the challenges for pandemic loss modeling highlighted here are precisely the challenges that the current generation of catastrophe models fails to address, ignores, or does not appropriately solve. Thinking more broadly about sovereign disaster risk finance, these are also fundamentally the societal impacts that a government must consider. Sovereign disaster risk financing solutions aim to support governments with their emergency losses after a natural disaster due to the additional need for health care, shelter, and food, and crucially the need to get their critical infrastructure up and running as soon as possible to mitigate indirect economic losses (for example, loss of tourism after a hurricane in the Caribbean). Typically, using the current modeling tools, sovereign disaster risk finance solutions quantify this risk by considering a fixed percentage of asset damages as representative of these government emergency costs—but we could do better.
Existing vulnerability and loss modules in catastrophe modeling frameworks are location specific and ignore the spatial and temporal connections between assets, economies, and people at risk. There is an ever-increasing conversation about critical infrastructure, network modeling, and the need to think about the broader role an individual risk can have in the system. This “next generation” of vulnerability modeling is a must in our globally connected world.
Photo Credit: World Bank / Henitsoa Rafalia
Financial Resilience Around the World | Blog Series
- Financial Resilience Around the World: Global Risk Financing Facility
- Three Ways Lesotho's Past Experience with Disasters Strengthen COVID-19 Response
- Three Steps to Help Albania Withstand the Financial Impacts of Disasters and Crises
- How Burkina Faso is Leveraging a Credit Guarantee Scheme to Help SMEs Weather the COVID-19 Economic Crisis